Netflix, Spotify and the evolution of recommender algorithms
Posted on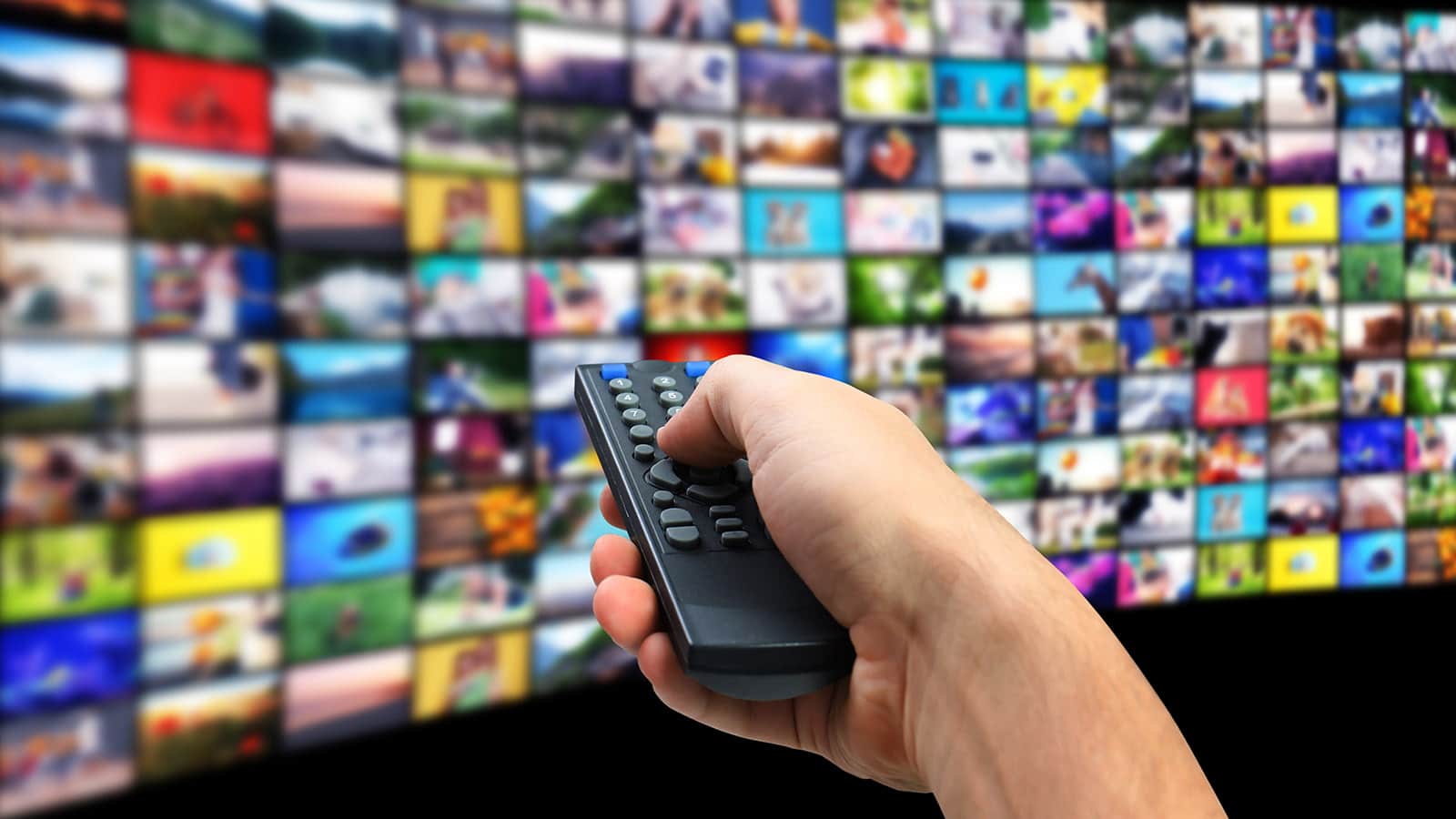
In today’s lightning-fast world, people are generating more data than ever before. Every mouse click, every search, every swipe on social media contributes an immense amount of information about behavior and preferences. Meanwhile, many thousands of movies, series and music tracks are available on online platforms such as Spotify and Netflix. How do you make optimal use of data on behavior and preferences? How do you help users make choices in the ocean of content? Welcome to the world of recommender algorithms!
In the latest episode of the JADS Data Dates podcast, we discuss the fascinating world of these recommender systems. Together with Martijn Willemsen, associate professor at JADS and researcher in the field of recommender systems, we highlight different aspects.
The history of recommender systems.
Recommender systems are not new; they arose from the need to help users find the right information in an ever-expanding digital world. It all started more than 25 years ago, when the Internet emerged and people were looking for ways to find relevant information. This led to the first forms of recommender systems in newsgroups and movie suggestions. In the 1990s, with the rise of e-commerce, Amazon began using recommender systems. They introduced “collaborative filtering,” one of the fundamental approaches to recommender systems. The idea behind this method is to make recommendations based on the preferences and interests of other users. The assumption is that if person A has similar tastes to person B, chances are that the items that person B liked will also be liked by person A. This is discussed in the podcast as the “People like you also liked” principle.
Content-based recommendations: A deeper dive into content
Whereas Collaborative Filtering leverages the social connections between users, Content-Based Recommendations, is all about the content of the items. For example, if a person likes sci-fi movies and thus watches a lot of them on Netflix, she encourages the algorithm to recommend similar content. Spotify, in turn, bases its recommendations on properties of songs; they analyze the audio characteristics of music and make recommendations based on properties such as energy, mood and danceability.
The importance of evaluation and diversity
A crucial topic touched on in the podcast is the evaluation of these recommender algorithms. How do you determine if a recommendation was actually helpful to the user? Various techniques, such as A/B testing and user research, are used to measure performance. In addition, the importance of diversity in recommendations is discussed. A good recommender system does not limit itself to a certain type of recommendation, but rather offers variety to allow the user to discover new things. This prevents users from slipping into a so-called “filter bubble,” where they are only exposed to content that matches their existing interests, which may cause them to encounter less diverse perspectives.
The future: machine learning and deep learning
The rise of machine learning and deep learning is presenting plenty of new opportunities for recommender systems. These technologies make it possible to identify complex patterns in user behavior and content characteristics. For example, neural networks can process vast amounts of data to make more accurate recommendations. This is mentioned as an exciting new area in the podcast, with the potential to take recommendations to the next level.
Dive into the world of recommendations
Are you curious about recommender algorithms and their profound impact on how we consume content and make choices online? Then listen to the JADS Data Dates podcast!
NB. The podcast is Dutch language only!